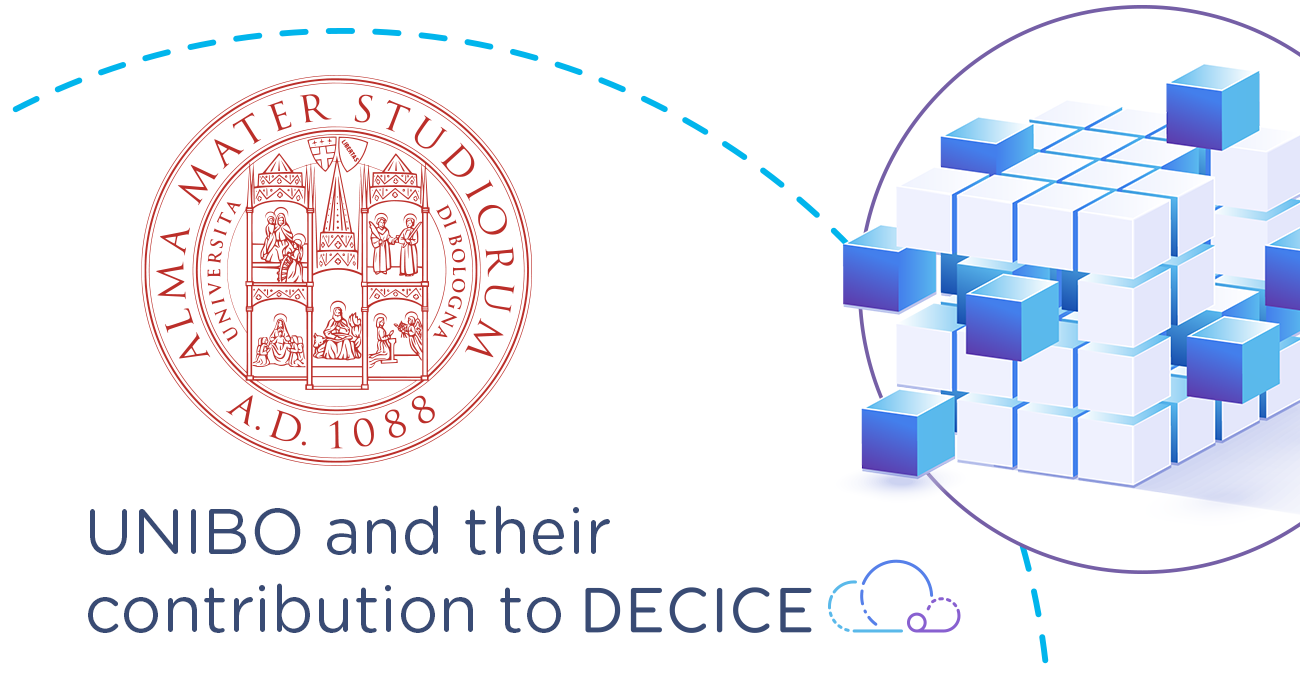
Let’s meet the brains behind the DECICE data-driven AI digital twin
Almost 1000 years old, the University of Bologna (UNIBO) is known as the oldest University in the western world. Nowadays, UNIBO is one of the most important institutions of higher education across Europe and the second largest university in Italy, with 11 Schools, 33 Departments, and about 84.000 students. The UNIBO team in the DECICE project is composed of researchers from the ECS Laboratory of the DEI department, which focuses on efficient cyber-physical system design. The group participating in the DECICE project is led by Prof. Andrea Bartolini. Andrea Bartolini’s research activities in recent years have investigated the creation of data-driven AI models of computing resources and their integration with a holistic monitoring system of computing clusters[1]. Early works have focused on the development of prediction models for the job’s energy and power consumption based on random forest ML models[2]. Recent works have proven that semi-supervised ML models are suitable for learning the complex relationship between performance metrics and run-time parameters and detecting anomalies in computing resources[3]. This model can be embedded in decision-making problems to prune suboptimal regions of the solution space effectively[4].In DECICE, UNIBO will combine the above-mentioned techniques into a data-driven AI digital twin of the computational resources serving to optimize the computing continuum.
In addition to that, Francesco Barchi, assistant professor in the ECS laboratory, will lead the prototyping of DECICE technologies to manage drone fleets in the environmental emergency response use case.
Author: Andrea Bartolini (UNIBO)
Reference
[1] A. Borghesi, A. Burrello and A. Bartolini, “ExaMon-X: a Predictive Maintenance Framework for Automatic Monitoring in Industrial IoT Systems,” in IEEE Internet of Things Journal, doi: 10.1109/JIOT.2021.3125885.
[2] Borghesi, A., Bartolini, A., Lombardi, M., Milano, M., Benini, L. (2016). Predictive Modeling for Job Power Consumption in HPC Systems. In: Kunkel, J., Balaji, P., Dongarra, J. (eds) High Performance Computing. ISC High Performance 2016.
[3] Andrea Borghesi, Andrea Bartolini, Michele Lombardi, Michela Milano, Luca Benini,A semisupervised autoencoder-based approach for anomaly detection in high performance computing systems, Engineering Applications of Artificial Intelligence, Volume 85,2019, Pages 634-644, 2019
[4] Michele Lombardi, Michela Milano, Andrea Bartolini, Empirical decision model learning, Artificial Intelligence, Volume 244, 2017, Pages 343-367, ISSN 0004-3702.
Links
Keywords
AI | Digital Twin | computing continuum | holistic monitoring system | computing clusters